The Equation Worth $1T
- Sam Wocks
- Mar 7, 2024
- 4 min read
Updated: Mar 26, 2024

This unassuming equation has quietly revolutionized the way we approach risk and uncertainty. Originating from physicists and mathematicians, this formula has become the backbone of modern finance, spawning multi-trillion dollar industries. Yet, despite its profound impact, few outside the realm of quantitative finance grasp the true significance of this equation and the story behind its creation.
Origin
The story of this equation begins with a young French mathematician named Louis Bachelier, who, at the dawn of the 20th century, stumbled upon a curious analogy between the seemingly chaotic movements of stock prices and the erratic motion of particles suspended in fluid, known as Brownian motion.
Bachelier's insights laid the foundation for the concept of the "random walk," a theory that would remain largely overlooked for decades, until a chain of remarkable events catapulted it to the forefront of financial innovation. This idea, laid the foundation for the modern understanding of financial markets as complex, adaptive systems.
Despite the groundbreaking nature of Bachelier's work, his ideas languished in obscurity for decades, largely ignored by the financial establishment. However, later a trio of economists would resurrect Bachelier's ideas and set the stage for a revolution in finance.
The Black-Scholes-Merton Revolution
In 1973, Fischer Black, Myron Scholes, and Robert Merton, a trio of economists and mathematicians, published a groundbreaking paper that would come to be known as the Black-Scholes-Merton (BSM) model. Building on the insights of Bachelier, the BSM model provided a precise mathematical formula for pricing options.
For those new to options, they're a type of financial derivative that gives investors the right, but not the obligation, to buy or sell an asset at a predetermined price in the future. Here's the basic 4 types, and when they each make and lose money:
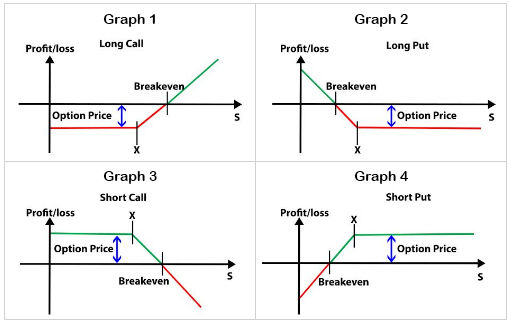
If you'd like to play around with option payoff values for your specific needs and variables, click the Google Colab icon below for (free) Python code!
Click this icon to see the code
The Black-Scholes-Merton (BSM) model was a game-changer, providing a rigorous, scientific approach to options pricing that had previously been the domain of gut instinct and market sentiment. By taking into account factors such as the underlying asset's price, volatility, and time to expiration, the BSM model allowed traders to calculate the fair value of an option with unprecedented accuracy, paving the way for the explosive growth of the derivatives market.
The equation is a testament to the power of mathematics to describe and predict the behavior of complex systems. This elegant formula would revolutionize the world of finance and spawn a multi-trillion dollar industry.
The Rise of Quantitative Hedge Funds
As the BSM model gained traction on Wall Street, a new breed of investment firm began to emerge, one that would come to be known as the quantitative hedge fund. These firms, led by mathematicians, physicists, and computer scientists, used advanced statistical models and computational techniques to identify patterns and anomalies in the market, seeking to exploit inefficiencies and generate outsized returns for their investors.
One of the most successful of these firms was Renaissance Technologies, founded by mathematician Jim Simons in 1978. Simons and his team of quantitative analysts, known as "quants," developed sophisticated algorithms that could process vast amounts of data and identify subtle patterns in the market, allowing them to make highly profitable trades with remarkable consistency.
They returned 66% per year... for decades...
At that rate of growth, $100 in 1988 would be worth $8.4B today.
At the heart of Renaissance's approach was the use of machine learning techniques, particularly a class of algorithms known as Hidden Markov Models (HMMs). These models, which had originally been developed for applications in speech recognition and bioinformatics, allowed Renaissance to uncover hidden states in the market, capturing the complex dependencies and relationships that drove asset prices.
Here's a simple example of HMMs in practice, with regimes that predict Bull (going up), Bear (going down), and Neutral (going sideways) market regimes. These regimes, and more complex versions, can be used as a strong foundation for developing investing strategies.

Click this icon to see the code
The success of firms like Renaissance demonstrated the immense potential of quantitative, data-driven approaches to investing, and soon, other firms began to follow suit. Today, quantitative hedge funds are a dominant force in the financial world, using advanced mathematical models and cutting-edge computational techniques to navigate the complexities of the market and deliver superior returns to their investors.
The Future of Quantitative Finance
As we move into the 21st century, the role of mathematics, physics, and computer science in finance shows no signs of diminishing. On the contrary, the rise of big data, artificial intelligence, and machine learning has only accelerated the pace of innovation, opening up new possibilities for quantitative analysis and data-driven decision making.
The story of how a physics equation sparked a trillion-dollar revolution in finance is a testament to the power of the ability to see connections across seemingly disparate fields. From the random walk of Brownian motion to the elegant Black-Scholes-Merton model, the history of quantitative finance is a story of brilliant minds coming together to tackle some of the most complex and challenging problems in the world of investing.
Precession AI
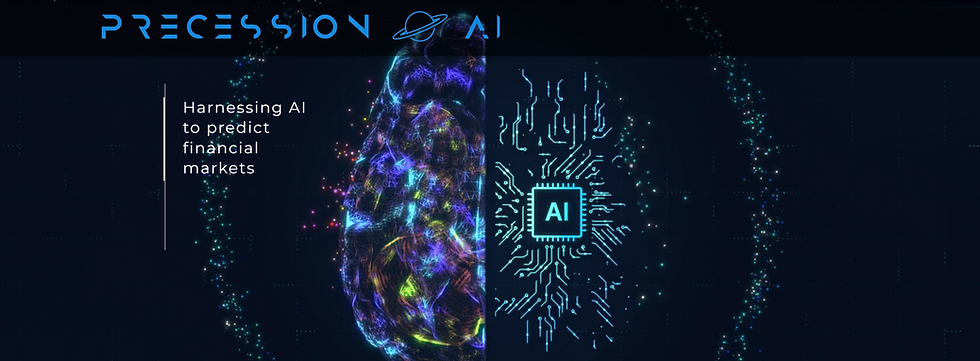
At Precession AI, we began with a mission:
What if there was a way to leverage state of the art Artificial Intelligence to unlock patterns in financial markets that have remained elusive to traditional techniques?
The approach involves sophisticated algorithms capable of digesting vast datasets, including historical market data, global news, and social media trends, to reveal hidden market patterns that are not explainable by traditional economic logic. These statistical anomalies are difficult to detect yet not random. These 'ghost patterns' unlock a novel way to predict stock movements, and the results speak for themselves:
In initial tests, we've seen 3 AI strategies achieve astonishing 1 yr. returns
From 20-Feb-2023 to 20-Feb-2024
199.5% - on SPDR
630.8% - on TSLA
16,656,072.3% - on BIT/USD
To put these figures into perspective, the S&P 500 ETF returned 21.9% over the same period. The AI generated strategy profits were an incredible
9.1x, 28.8x, and 760.6x higher, respectively.
GET IN TOUCH
If you're interested in leveraging Precession AI's cutting-edge strategies for your investments, we'd love to hear from you.
Also, join our community to stay ahead of the curve with the latest insights on AI investing. Receive all news, updates, and articles directly to your inbox.
Comments